Build Land Use Segmentation AI for High-resolution Drone Photos
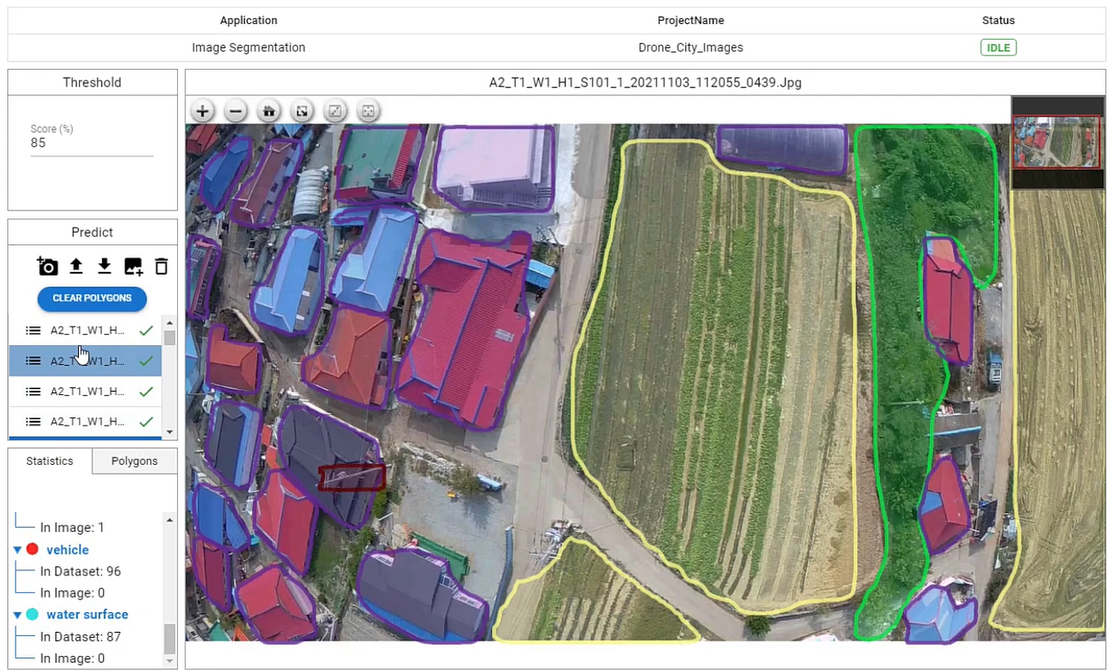
Build your own HIGH RESOLUTION image analysis machine learning models without coding! - DeepBlock.net
This video shows how to build land use segmentation AI that can identify various types of lands in ultra-high-resolution drone photos, allowing you to see the increase or decrease in land for various purposes and the area of each type of land. Create your own machine vision model without coding and use it to automate your ultra-high-resolution image analysis.
If you want to try the machine learning project shown in the video, check this link.
In this video, we will build and use an AI model using Deep Block to identify land use from high-resolution drone photos. Deep Block is a no-code machine learning software, and a web platform that allows users to create and use their own machine vision models without coding. In this video, we will implement an image segmentation model to identify and segment ground in rural areas of Korea. We already have almost all the training data ready to train our model. As you can see in the video, DeepBlock.net provides annotation tool that can prepare training data to distinguish farmland, buildings, vehicles from drone photographs. As you can see in the video, if you diligently draw a polygon from a photo for each class, the AI model can be trained based on the polygons of each class.
But the capabilities of Deep Block don't end there. Not only can it analyze small drone photos, but it also has the capacity to handle ultra-high-resolution GEOTIFF images, stitched drone photos, and even aerial photos taken at altitudes of 500m or higher. As demonstrated in the video, a data provider, South Korean company had to divide and distribute their drone images online simply because they lacked a specialized machine learning software and software technology to process such large volumes of data.
For those fortunate enough to harness the power of Deep Block, uploading ultra-high-resolution GEOTIFF aerial images or satellite photographs is a breeze. The software fully supports GEOTIFF and JP2 formats, ensuring a seamless integration of your data.
Once your training data is prepared for the image segmentation model, it's time to train your model. Simply set the desired number of Epochs, such as 50, and hit the coveted "Train" button. It's important to note that due to the size of the training data for this project, the training process may take some time. So, while your model is being trained, feel free to engage in other tasks or explore the Project Store of Deep Block, where pre-trained projects are readily available including this project.
Once the training of your cutting-edge land use segmentation model is complete, the real magic happens. Upload new images and witness the power of the "PREDICT" button. After a short period, you will be able to effortlessly identify various structures on the ground within the drone images. And for those seeking even faster inference speed, be sure to reach out to us through our website.
In addition, images in GEOTIFF and JP2 formats can be used in inference mode, and the inference results can be exported in GEOJSON format when utilizing GEOTIFF images. As demonstrated, the machine learning model excels at identifying land use on the ground, opening up a world of possibilities. If you're eager to experience the potential of this remarkable model for yourself, don't hesitate to visit DeepBlock.net now.