How to train accurate AI models for Building Segmentation?
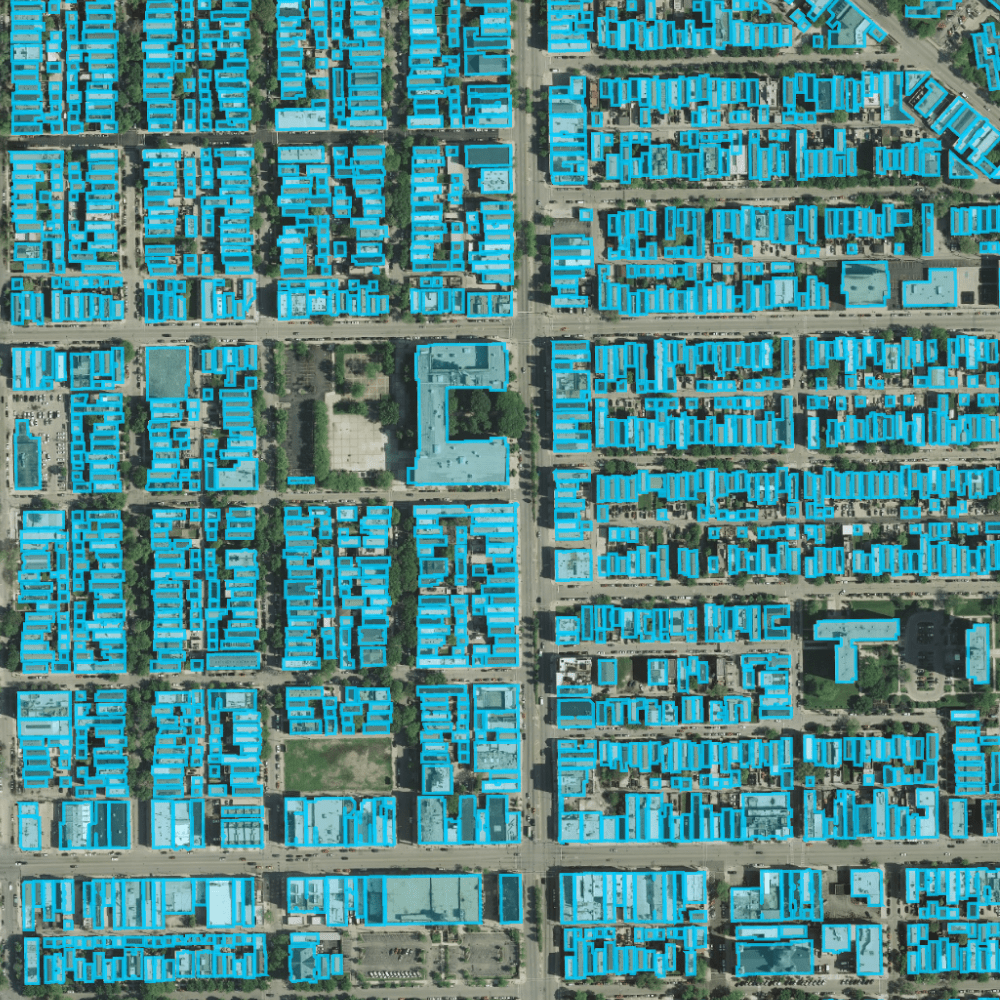
In today's era of burgeoning technology, the integration of artificial intelligence (AI) and geospatial analysis has opened unprecedented avenues for extracting intricate insights from satellite imagery. Among the myriad applications of AI in remote sensing, image segmentation stands out as a powerful technique, particularly when applied to building identification and analysis. In this article, we delve into the realm of building segmentation, exploring its significance, challenges, and the revolutionary role played by Deep Block, an AI-powered geospatial analysis platform.
What is Building Segmentation?
Understanding Image Segmentation: Image segmentation is a sophisticated computer vision technique that involves dividing an image into distinct regions, each corresponding to a particular object or category. This process facilitates the identification and differentiation of various elements within an image, enabling accurate object recognition and analysis. In satellite imagery, image segmentation becomes a crucial tool for understanding and categorizing the complexities of landscapes, from urban environments to agricultural fields.
The Significance of Building Segmentation: Building segmentation, a specific application of image segmentation, focuses on isolating and delineating structures within satellite imagery. In urban planning, disaster management, and infrastructure development, the ability to accurately identify and analyze buildings provides invaluable insights. Building segmentation aids in a growing range of use cases:
- Urban Planning and Development: Building segmentation plays a pivotal role in urban planning by accurately identifying and delineating individual structures within a city. This information assists in zoning regulations, land-use assessments, illegal construction detection, and infrastructure development planning.
- Disaster Management: In the aftermath of disasters such as earthquakes or floods, building segmentation helps assess damage and allocate resources efficiently. Rapid identification of collapsed structures and affected areas aids in directing rescue operations and recovery efforts.
- Environmental Impact Assessment: Building segmentation contributes to environmental impact assessments by identifying changes in land usage over time. Detecting new construction, changes in building density, or unauthorized developments assists in monitoring and mitigating ecological impacts.
- Infrastructure Maintenance: Building segmentation aids in routine infrastructure maintenance by identifying deteriorating structures, assessing maintenance requirements, and prioritizing repairs. This ensures the optimal allocation of resources for upkeep.
- Real Estate Market Analysis: In real estate, building segmentation assists in analyzing property values, housing density, and property characteristics. This information aids in property valuation, market trend analysis, and urban expansion predictions.
- Energy Efficiency Analysis: Building segmentation aids in assessing energy efficiency by identifying building materials, roof types, and insulation patterns. This information informs energy audits and retrofitting strategies for improved sustainability.
- Transportation Planning: In transportation planning, building segmentation helps analyze traffic flow, parking demand, and road infrastructure needs. This information contributes to efficient transportation network design.
- Renewable Energy Deployment: Building segmentation assists in identifying suitable rooftops and surfaces for solar panel installation. This information supports the deployment of renewable energy infrastructure.
- Archaeological Research: In archaeological research, building segmentation helps identify and locate ancient structures and settlements buried beneath modern landscapes. This aids in understanding historical urban development.
Challenges in Large-Scale Building Segmentation: While the potential benefits of building segmentation are substantial, the challenges associated with accurate identification on a large scale are equally formidable. Large geospatial datasets contain vast amounts of information, introducing complexities like varying lighting conditions, diverse architectural styles, and intricate backgrounds. Traditional manual methods of building segmentation are not only time-consuming but also prone to inaccuracies, limiting their utility in practical applications.
Deep Block: Revolutionizing Building Segmentation.
This is where Deep Block emerges as a game-changer. Deep Block, an AI-powered geospatial analysis platform, employs cutting-edge image segmentation techniques to deliver accurate and efficient building identification on a large scale. With its robust AI pipeline and intuitive interface, Deep Block overcomes the challenges of building segmentation, making the process faster, more accurate, and highly scalable.
Deep Block in Action: A compelling testament to Deep Block's capabilities can be observed in a recently conducted experiment. By leveraging Deep Block's image segmentation techniques, an intricate urban landscape was segmented into distinct buildings. The process, which would traditionally take hours or even days, was completed in a matter of minutes. This speed and accuracy significantly amplify the practicality of building segmentation in time-sensitive scenarios.
Accurate Model Training: The key to accurate building segmentation lies in training reliable AI models. Deep Block's platform allows users to train custom machine learning models using their own labeled datasets. This capability is particularly crucial in building segmentation, where architectural diversity and complex surroundings demand a high level of model customization. Deep Block's extensive library of pre-trained models further expedites the training process, enabling users to fine-tune models to specific contexts.
High-Speed Inference: Once trained, the AI models deployed within Deep Block showcase their true power during the inference phase. Deep Block's AI pipeline facilitates rapid model execution, allowing users to perform inference on large-scale geospatial datasets with remarkable speed. This speed is critical for real-time or near-real-time applications such as disaster response, infrastructure monitoring, and urban planning.
In the world of geospatial analysis, image segmentation stands as a technological milestone that transforms complex imagery into actionable insights. Deep Block, with its powerful AI-driven platform, brings this technique to the forefront of building segmentation in satellite imagery. By allowing users to train customized models and execute high-speed inferences, Deep Block not only surmounts the challenges of accurate building identification but also unlocks the potential for real-time analysis on a large scale. As urban landscapes evolve and disaster preparedness gains prominence, the fusion of AI and image segmentation within Deep Block emerges as a beacon of innovation, promising a more informed and responsive future.