Deep Block releases Tiling for Enhanced Detection in High-Res Images.
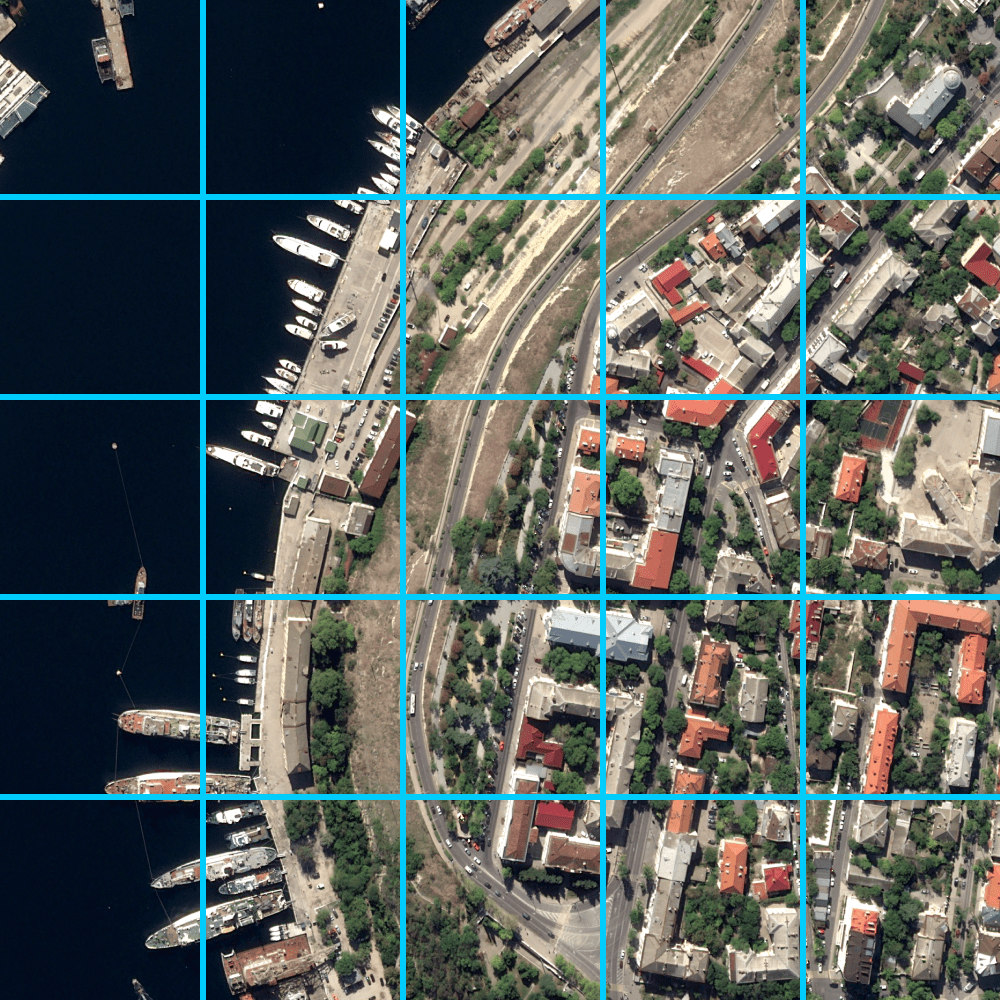
The realm of image analysis has experienced a monumental transformation with the rise of deep learning technologies. These breakthroughs have found applications in various specialized industries, including facial recognition in social media, object detection in autonomous driving, satellite image analysis, plant disease detection, and cancer cell identification. The underpinning of these advancements lies in the diverse array of image analysis techniques, ranging from image classification to object detection, segmentation, object tracking, and change detection. However, as we venture into the territory of high-resolution images, a new set of challenges emerges.
High-resolution images, characterized by their astonishing detail and clarity, pose unique computational challenges. Conventional deep learning models often struggle to process these images efficiently, hampering their utility in applications that require rapid and accurate object detection.
To overcome these obstacles, Deep Block released a patented tiling feature. This feature aims to revolutionize object detection in high-resolution images by enhancing its accuracy and efficiency.
Challenges Addressed: The primary challenge addressed by this invention is the accurate and efficient detection of objects in high-resolution images. Existing object detection techniques face numerous hurdles when working with images of such scale and intricacy. These challenges manifest as reduced accuracy, prolonged processing times, and the inability to effectively manage overlapping objects. Deep Block's patented tiling technology's objective is to elevate detection accuracy while preserving computational efficiency.
Method Overview: The groundbreaking method proposed in this patent incorporates several pivotal steps to realize precise and efficient object detection in high-resolution images:
- Tiling for Enhanced Segmentation: The cornerstone of our approach lies in tiling. The input image is meticulously divided into a set of tiles using a sophisticated tile division unit. These tiles fall into two categories: normal and auxiliary tiles. Normal tiles encompass the entire image area, while auxiliary tiles include boundary lines from normal tiles. This strategic segmentation facilitates precise object detection, even for objects divided by the boundaries of normal tiles.
- Parallel Object Detection: With the tiles in place, a first object detection unit takes the reins, operating in parallel to identify target objects. Regardless of whether the tiles are normal or auxiliary, each is employed to facilitate comprehensive object recognition. This multi-pronged approach ensures a thorough examination of the image, enabling accurate detection while accounting for overlapping objects.
- Tile Merging for Comprehensive Insight: Following the initial object detection, the tile merge unit steps in to process the identified objects from different tiles, expertly merging them as necessary. This crucial step harmonizes the detection results and minimizes redundancy, a common issue in object detection due to overlapping detections.
- Redundant Detection Refinement: To fine-tune the detection results, the second object detection unit enters the scene, focusing on the merged tiles. This unit effectively deals with three types of redundancy:
- First-type redundancy: Occurs when an object is detected in auxiliary tiles but not in normal tiles.
- Second-type redundancy: Arises when one tile detects part of an object, while another tile detects the entire object.
- Third-type redundancy: Happens when both auxiliary and normal tiles identify the entire object.
Each type of redundancy is systematically managed, ensuring that the final detection is both accurate and free of redundant detections.
Advantages: The proposed method offers a myriad of advantages over conventional object detection approaches when applied to high-resolution images:
- Enhanced Accuracy: By strategically dividing images into tiles and managing overlapping detections, the method achieves superior accuracy in object detection.
- Efficiency through Parallel Processing: The parallel processing of tiles ensures efficient detection, even in large high-resolution images.
- Adaptability to Various Resolutions: The method can seamlessly adapt to different image resolutions, making it versatile for a wide range of applications.
- Redundant Detection Handling: The ability to effectively handle redundant detections sets this method apart, ensuring that final detection results are both precise and devoid of duplicates.
The innovative object detection method using tiling represents a monumental leap in the quest for accurate object detection in high-resolution images. By thoughtfully segmenting images into tiles and efficiently processing these tiles, this method surmounts the limitations of traditional approaches, offering unparalleled accuracy and efficiency. In an era where high-resolution imagery is increasingly prevalent, this technology paves the way for more effective and accurate computer vision applications across a multitude of domains.